A small tolerance value indicates that the variable under consideration is almost a perfect linear combination of the independent variables already in the equation and that it should not be added to the regression equation. Though the literature on ways of coping with collinearity is extensive relatively little effort has been made to clarify the conditions under which collinearity affects estimates developed with multiple regression analysisor how pronounced.
How To Interpret A Collinearity Diagnostics Table In Spss
Remaining predictors is very high.
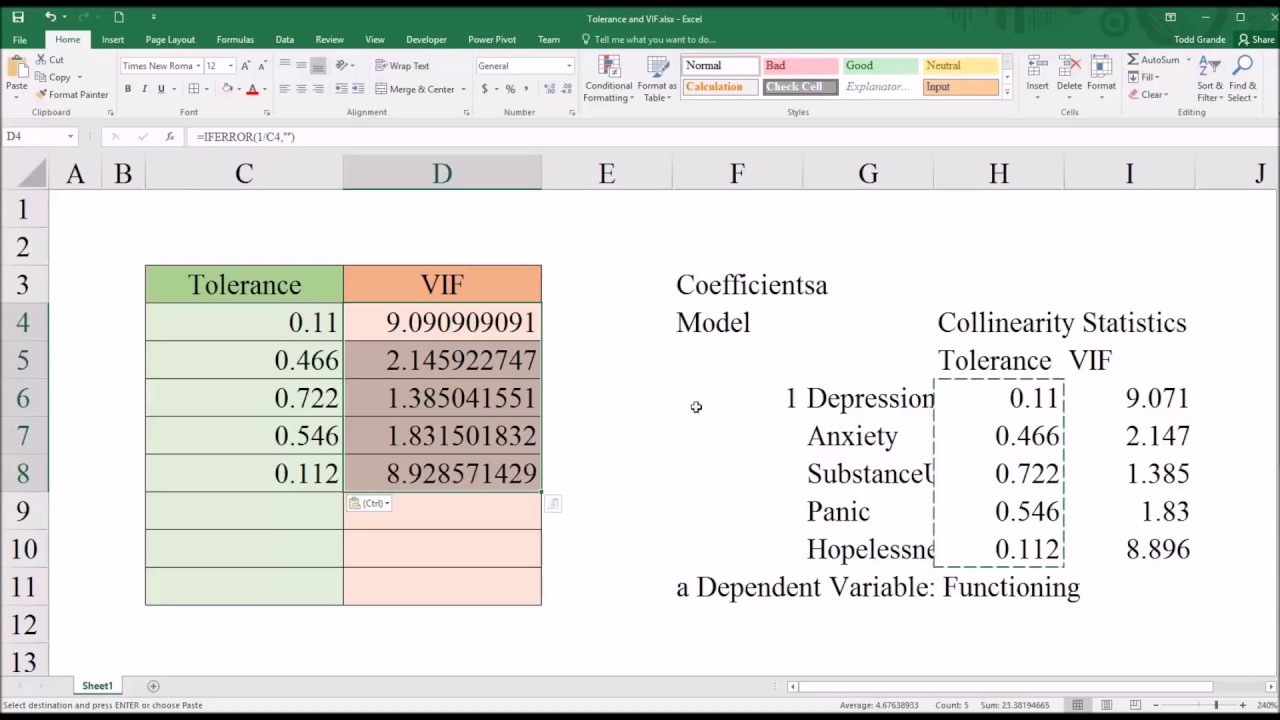
Collinearity statistics spss interpretation. The tolerance is an indication of the percent of variance in an independent that cannot be accounted for by the other independent variables hence very small values indicate that an independent variable is redundant. One is tolerance which is simply 1 minus that R2. SPSS automatically does a tolerance analysis and wont enter the regression model any variable.
A rule of thumb is to label as large those condition indices in the range of 30 or larger. How to Test for Multicollinearity in SPSS. The most common summary statistic for evaluating collinearity is tolerance.
The collinearity diagnostics confirm that there are serious problems with multicollinearity. Multicollinearity in regression analysis occurs when two or more predictor variables are highly correlated to each other such that they do not provide unique or independent information in the regression model. Tolerance is a measure of collinearity reported by most statistical programs such as SPSS.
The variables tolerance is 1-R2. To see if the data meets the assumption of collinearity you need to locate the Coefficients table in your results. The tolerance value for a particular predictor in a particular model is 1 - R² where the R² is obtained using that predictor as a criterion and all others as predictors.
If the degree of correlation is high enough between variables it can cause problems when. First in the Coefficients table on the far right a Collinearity. Set up your regression as if you were going to run it by putting your outcome dependent variable and predictor independent variables in the appropriate boxes.
Yet correlated predictor variablesand potential collinearity effectsare a common concern in interpretation of regression estimates. T able 4 and Table 5 present the collinearity statistics. Through the estimation of interpretation of coefficients from a wide variety of logistic.
BMI Unstandardized coefficients used in the prediction and interpretation standardized coefficients used for comparing the effects of independent variables Compared Sig. In thinking about it that only thing I can think in how it addresses that collinearity issue is that it percolates through to the actual regression and reduces the effect this collinearity has on the dependent var by altering x1 and x2s coefficients while decreasing x1 and x2s numerical values by essentially adding two more constants to the regression so instead. Several eigenvalues are close to 0 indicating that the predictors are highly intercorrelated and that small changes in the data values may lead to large changes in the estimates of the coefficients.
It is advisable to additionally include the collinearity diagnostics and the Durbin-Watson test for auto-correlation. If the VIF value is greater than 10 or the Tolerance is less than 01 then you have concerns over. The most extreme example of this would be if you did something like.
Statology Study is the ultimate online statistics study guide that helps you understand all of the core concepts taught in any elementary statistics course and. Collinearity is spotted by finding 2 or more variables that have large proportions of variance 50 or more that correspond to large condition indices. The second is VIF the variance inflation factor which is simply the reciprocal of the tolerance.
Multicollinearity is where more than. The Casewise Diagnostics table is a list of all cases for which the residuals size exceeds 3. Collinearity Diagnostics emerge from our output next.
How to interpret a Collinearity Diagnostics table in SPSS. There is no evident problem with collinearity in the above example. The table is part of the calculation of the collinearity statistics.
Discovering Statistics Using SPSS 3rd. If the option Collinearity Diagnostics is selected in the context of multiple regression two additional pieces of information are obtained in the SPSS output. Abstract Multicollinearity occurs when the multiple linear regression analysis includes several variables that are.
Interpretation of Test Results Output Multicollinearity Based on the Coefficients Output - collinearity Statistics obtained VIF value of 1812 meaning that the VIF value obtained is between 1 to 10 it can be concluded that there is no multicollinearity symptoms. Very low values of tolerance 1 or less indicate a problem. Collinearity is an association or correlation between two predictor or independent variables in a statistical model.
The field statistics allows us to include additional statistics that we need to assess the validity of our linear regression analysis. To fully check the assumptions of the regression using a normal P-P plot a scatterplot of the residuals and VIF values bring up your data in SPSS and select Analyze Regression Linear. We will not discuss this here because understanding the exact nature of this table is beyond the scope of this website.
. Here you will see the heading Collinearity Statistics under which are two subheadings Tolerance and VIF. Multicollinearity is a problem that occurs with regression analysis when there is a high correlation of at least one independent variable with a combination of the other independent variables.
Significantly correlated not on ly with the dependent variable but also to each. Collinearity is spotted by finding 2 or more variables that have large proportions of variance 50 or more that correspond to large condition indices. Collinearity statistics measure the relationship between multiple independent variables by giving a score for each independent.
Upon request SPSS will give you two transformations of the squared multiple correlation coefficients. A rule of thumb is to label as large those condition indices in the range of 30 or larger. Model.
Multicollinearity Test Example Using Spss Spss Tests
Testing For The Presence Of Multicollinearity In Spss Youtube
Collinearity Statistics Tolerance Test And Variance Inflation Factor Vif Download Table
Interpretation And Steps To Do Multi Collinearity Tests Deepa Enlighten
Understanding And Identifying Multicollinearity In Regression Using Spss Youtube
Multicollinearity Test Example Using Spss Spss Tests
How To Interpret A Collinearity Diagnostics Table In Spss
Testing Assumptions Of Linear Regression In Spss Statistics Solutions
Multicollinearity Test Example Using Spss Spss Tests
Multicollinearity Statistics With Spss Annmaria S Blog
Multicollinearity Statistics With Spss Annmaria S Blog
How To Interpret A Collinearity Diagnostics Table In Spss
How To Interpret A Collinearity Diagnostics Table In Spss
Multicollinearity Test Example Using Spss Spss Tests
How To Interpret A Collinearity Diagnostics Table In Spss
Multicollinearity Diagnostics How Are The Eigenvalues Calculated Cross Validated
Detection Of Multicollinearity Based On Collinearity Statistics Download Table
Posting Komentar